Math Fundamentals for Data Science and Machine Learning
Learn the mathematical pillars of data science and AI/ML.
About this learning path
Recognizing foundational math concepts in your data science career is key to understanding the underpinnings of many ML algorithms and models. Statistics, probability, and linear algebra form the backbone of data science and ML, enabling practitioners to extract meaningful insights from data, make informed decisions, and build robust models. Upon successful completion of this learning path, you’ll be equipped with essential math concepts, empowering you to navigate the complexities of data science and ML with precision and confidence.
By the end of this learning path, you’ll learn:
- Core principles, such as gradient descent and regression models, that are crucial for model formulation, training, and evaluation.
- Fundamental math concepts that underpin AI, empowering you to build and deploy AI solutions at a more profound and impactful level.
- How mastery of math concepts can help you improve your analytical and problem-solving skills, which are imperative to address challenges encountered in AI/ML projects.
This learning path is for you because…
- You’re a data science professional looking to better understand fundamental math concepts.
- You wish to become a better ML practitioner by understanding the role of probability, statistics, hypothesis testing, linear algebra, and calculus in ML.
- You work with data scientists, ML practitioners, or statisticians who frequently use mathematical models in their daily work.
- You’re an analyst, a project manager working with data science teams, or a software engineer interested in numerical computing.
Prerequisites
- Get Started with Anaconda course (optional)
- Basic Python proficiency (e.g., variables, loops, collections, and functions) and familiarity with library usage.
Facilitator bio:
Thomas Nield is the founder of Nield Consulting Group and Yawman Flight, as well as an instructor at University of Southern California. He enjoys making technical content relatable and relevant to those unfamiliar or intimidated by it. Thomas regularly teaches classes on data analysis, machine learning, mathematical optimization, and practical artificial intelligence. At USC he teaches AI System Safety, developing systematic approaches for identifying AI-related hazards in aviation and ground vehicles. He's authored three books, including Essential Math for Data Science (O’Reilly) and Getting Started with SQL (O'Reilly).
He is also the founder and inventor of Yawman Flight, a company developing universal handheld flight controls for flight simulation and unmanned aerial vehicles. You can find him on Twitter | LinkedIn | GitHub | YouTube.
Questions? Issues? Join our Community page to get help.
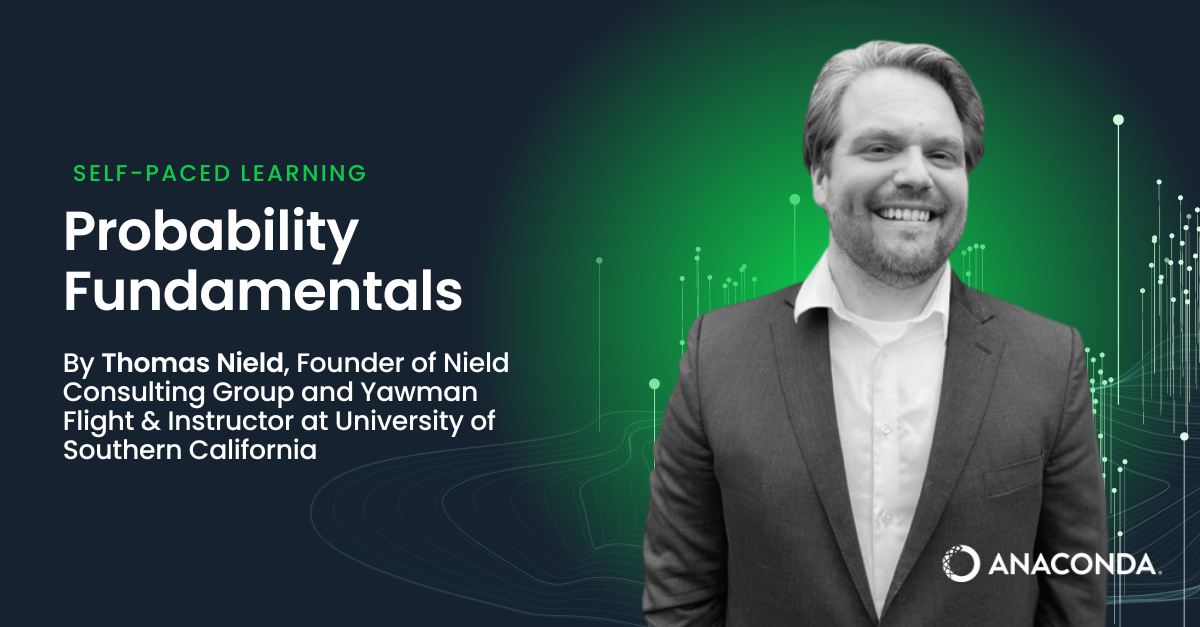
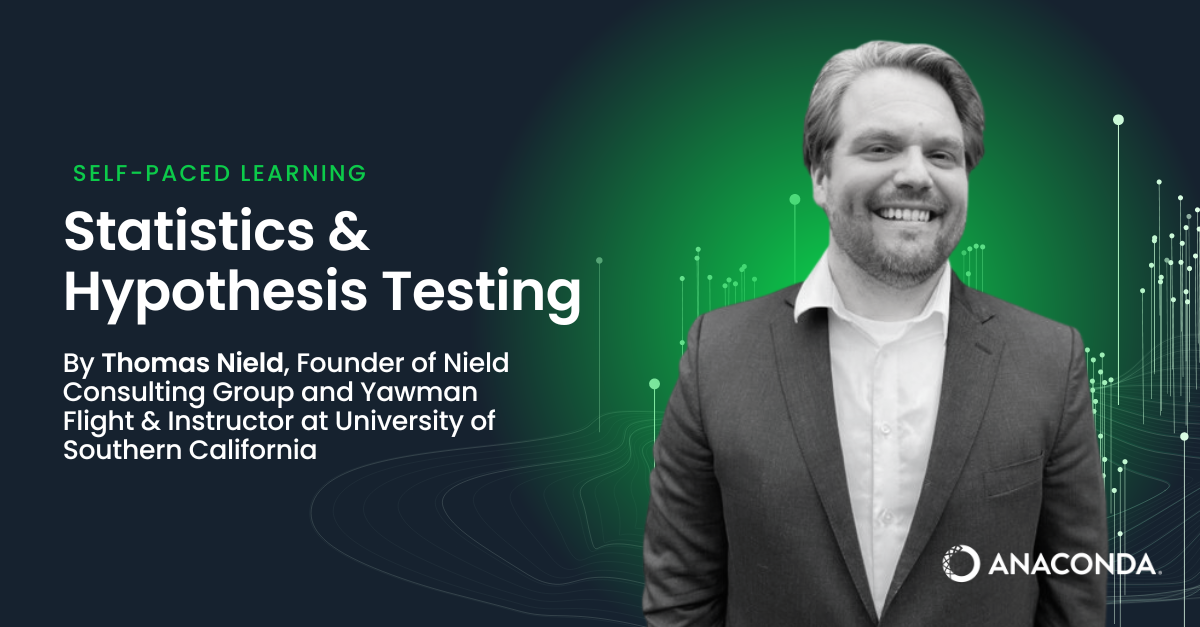
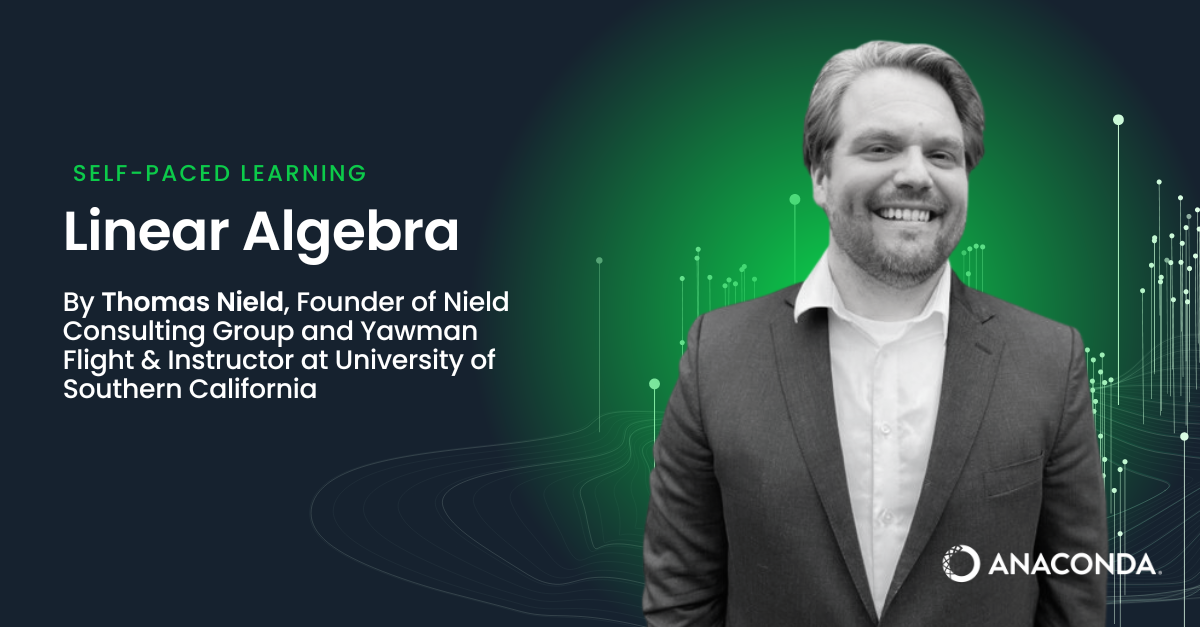
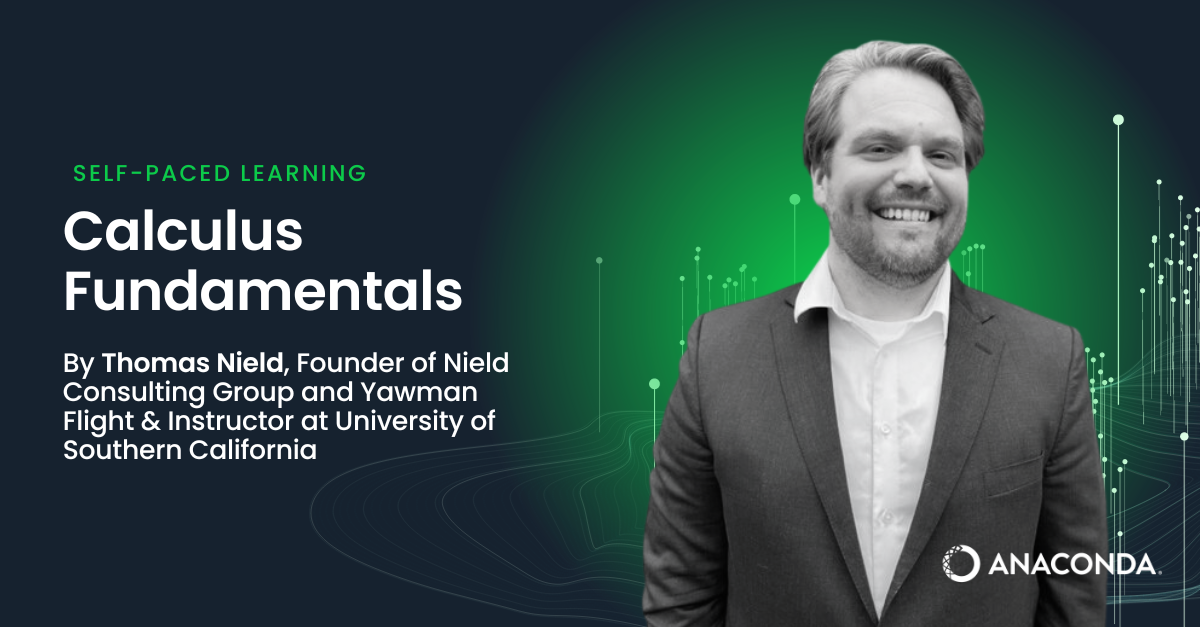